- Published on
Safety Data Communities and Types
- Authors
- Name
- Sean McGregor
- @seanmcgregor
I recently collaborated with 33 excellent co-authors on a position paper, "In House Evaluation Is Not Enough: Towards Robust Third-Party Flaw Disclosure for General-Purpose AI". My one sentence version of the paper is: "researchers need this pathway to disclose when there is a problem with an AI system in the world." Otherwise, bad things will needlessly happen to people. An element of the paper I am particularly proud of positions "flaw reporting" as covering a variety of "safety data" types -- each of which require distinctive evidence and process. These distinctions often produce unproductive arguments among digital safety practitioners hailing from some mixture of the computer security, product safety, trust and safety, and corporate risk teams.
Consider the following graphic from the paper:
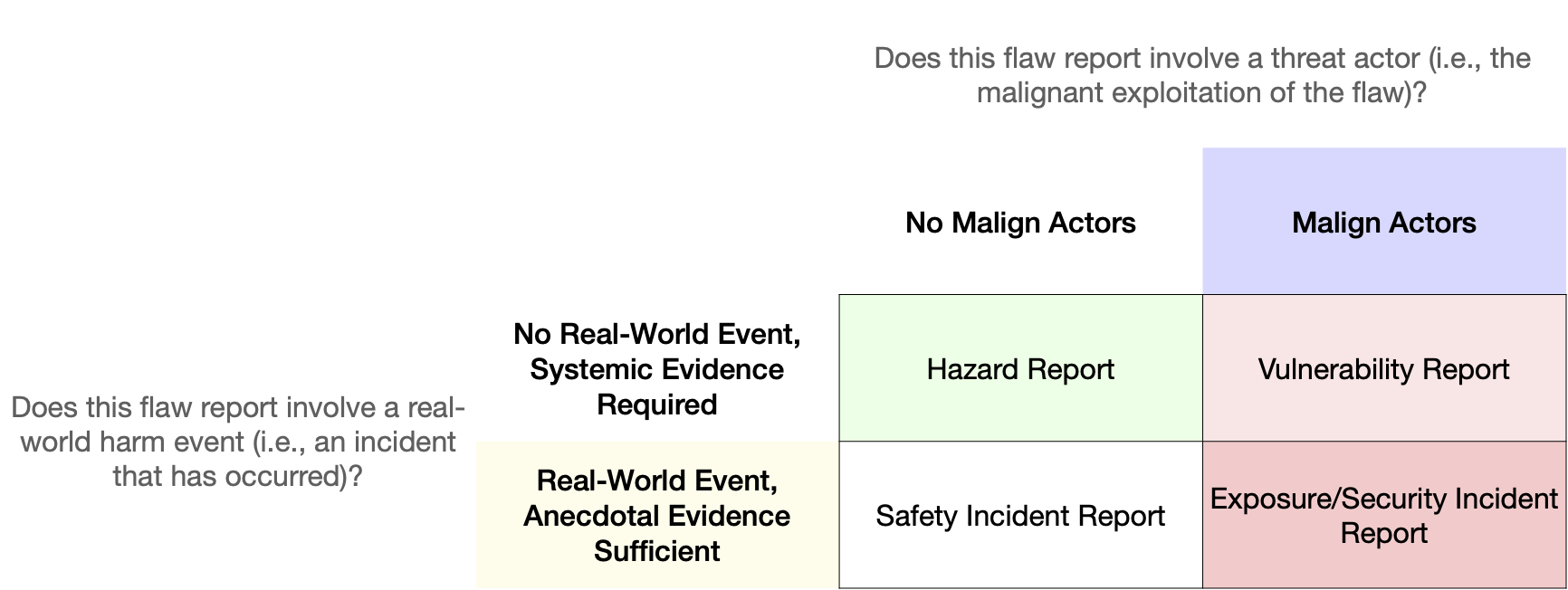
A matrix of AI safety data types broken out by dimensions separating the communities that trade in them (security vs product safety) and the types of evidence required.
Discussions often degenerate because researchers addressing the malign actors column (typically computer security researchers) do not operate from the presumptions of people researching hazards (most often people in the AI ethics community). Since safety only exists when a system is non-hazardous and non-vulnerable, these communities need to work together to make a safer digital ecosystem. But they also need to develop their own processes. This table reconciles terms from the AI Incident Database, OECD, MITRE, and AVID, among others, so we can better identify and communicate with one another while not insisting on the universal adoption of a single viewpoint on safety.
Where the columns differ by communities, the rows differ in the methodologies required to evidence the flaw. Incidents are events with strong evidence of harm potential. You don't need to run a statistical analysis...it happened! Conversely, if an event did not occur in the real world, system developers need statistical evidence. This is something we explored at DEF CON -- we didn't want "here is a bad thing the model did when I was trying to make it do bad things." This is easy to produce for any model. What we wanted was evidence the system was systematically hazardous or vulnerable.
I would encourage anyone looking to communicate with peers in digital safety data to first identify where they are operating from in this grid. Further, I would encourage separate research efforts to address the rows, columns, and cells of this table. In terms of process, organizations should then look to unify all these forms of flaw reporting and create comprehensive digital safety programs responsive to each of these report types.